Calculating price movements
By Nick Franks on Thursday, May 15th, 2025In my previous blog post, I explained how meaningful odds can be extracted from the betting_text column in the historic_runners tables. We will now discuss how these odds can be converted into a decimal price and compared again with the starting price to produce a price movement for each runner in a race.
The SQL script below builds a table called all_prev_race_price_movements. It holds information about each horse’s latest race for a specific type of race (such as turf, all-weather, etc.) and, where possible, details from the horse’s previous race of the same type.
The script then works out how the betting odds changed between the two races — basically, tracking if a horse was backed or drifted in the market.
The script returns not just the price movement but other pieces of useful data for the current and previous runs. Eg. finish_position, position_in_betting, unfinished, distance_won, distance_beaten, etc. (This data will be useful in the next blog post, where we will show how the table created can be use to analyse the data further).
Takeaways from the full SQL script below:
This setup allows you to:
- See how a horse’s market position changed from one race to the next.
- Spot if a horse was heavily backed (shortened in price) or was not fancied (ie. the price drifted).
- Compare races of the same type only, so the analysis is consistent.
Step-by-Step Breakdown
1. RankedRaces
- This section links together races and runners.
- It gives each race a ranking within its race type, with the most recent races ranked highest.
2. LastRaceTimes
- This picks out the most recent race for each race type.
- It helps make sure we only look at races up to the latest one — anything in the future gets ignored.
3. FilteredRaces
This is the main bit of the logic.
- It filters out races that happened after the most recent one.
- It ranks each runner’s races within that race type — so we can tell which was most recent run, which was next most recent run, and so on.
- It fetches details like the starting price, where the horse finished, etc.
- It also attempts to extract the opening odds from the betting summary text ( as covered in my previous blog post).
- It calculates a percentage chance (ie. probability) implied by the starting price.
4. ProcessedRaces
- Adds a calculation to convert the opening odds into a percentage.
- This allows us to compare the market expectation before the race with the actual SP (starting price).
Final Output (SELECT Statement)
The final section:
- Focuses on the most recent race for each horse and race type.
- Tries to link it to the previous race of the same type for the same horse.
- If no such race exists, the previous race columns are just left empty (NULL).
- Works out the percentage change from the opening price to the starting price — this shows whether the horse was backed in (price dropped) or drifted (price lengthened).
- The whole code below has the following criteria to return results for horses in races in the last 3 months, this can be changed as desired.
AND curr.scheduled_time >= DATE_SUB(CURRENT_DATE, INTERVAL 3 MONTH)
The results of this SQL can been seen by running a simple select:
SELECT * FROM all_prev_race_price_movements;
Full SQL Script:
DROP TABLE IF EXISTS all_prev_race_price_movements;
CREATE TABLE all_prev_race_price_movements AS
WITH RankedRaces AS (
SELECT
hr.scheduled_time,
hru.runner_id,
hr.race_id,
hr.race_type_id,
hr.handicap,
hru.starting_price,
hru.starting_price_decimal,
hru.position_in_betting,
hru.finish_position,
hru.unfinished,
hru.distance_won,
hru.distance_beaten,
hru.official_rating,
hru.betting_text,
RANK() OVER (PARTITION BY hr.race_type_id ORDER BY hr.scheduled_time DESC) AS race_type_rank
FROM historic_races hr
JOIN historic_runners hru USING (race_id)
), LastRaceTimes AS (
SELECT
race_type_id,
MAX(scheduled_time) AS last_race_time
FROM RankedRaces
WHERE race_type_rank = 1
GROUP BY race_type_id
), FilteredRaces AS (
SELECT
rr.scheduled_time,
rr.runner_id,
rr.race_id,
rr.race_type_id,
rr.handicap,
rr.official_rating,
rr.starting_price AS previous_starting_price,
rr.starting_price_decimal AS previous_starting_price_decimal,
rr.position_in_betting AS previous_position_in_betting,
rr.finish_position AS previous_finish_position,
rr.unfinished AS previous_unfinished,
rr.distance_beaten AS previous_distance_won,
rr.distance_beaten AS previous_distance_beaten,
rr.official_rating AS previous_official_rating,
rr.handicap AS previous_handicap,
rr.betting_text AS previous_betting_text,
-- Parse odds string
IFNULL(
REPLACE(
REGEXP_SUBSTR(
rr.betting_text,
'(?<=op )\\d{1,3}/\\d{1,3}|(?<=tchd )\\d{1,3}/\\d{1,3}|(?<=op )Evens|(?<=tchd )Evens'
),
'Evens',
'1/1'
),
'0/0'
) AS derived_previous_odds,
-- Cast to prevent divide by zero, using DECIMAL to avoid FLOAT deprecation
CASE
WHEN rr.starting_price_decimal > 0 THEN
CAST(CAST(100 AS DECIMAL(20,10)) / CAST(rr.starting_price_decimal AS DECIMAL(20,10)) AS DECIMAL(20,10))
ELSE NULL
END AS previous_SP_pct,
ROW_NUMBER() OVER (PARTITION BY rr.runner_id, rr.race_type_id ORDER BY rr.scheduled_time DESC) AS race_rank
FROM RankedRaces rr
JOIN LastRaceTimes lrt ON rr.race_type_id = lrt.race_type_id
WHERE rr.scheduled_time <= lrt.last_race_time
), ProcessedRaces AS (
SELECT *,
-- Convert odds string to decimal price percentage using DECIMAL
CASE
WHEN REGEXP_LIKE(derived_previous_odds, '\\d+/\\d+') AND
CAST(SUBSTRING_INDEX(derived_previous_odds, '/', -1) AS DECIMAL(20,10)) > 0
THEN CAST(
CAST(100 AS DECIMAL(20,10)) / (
CAST(SUBSTRING_INDEX(derived_previous_odds, '/', 1) AS DECIMAL(20,10)) /
CAST(SUBSTRING_INDEX(derived_previous_odds, '/', -1) AS DECIMAL(20,10)) +
CAST(1 AS DECIMAL(20,10))
)
AS DECIMAL(20,10)
)
ELSE NULL
END AS previous_opening_price_pct
FROM FilteredRaces
)
SELECT
curr.runner_id,
curr.race_type_id,
curr.scheduled_time AS current_race_time,
curr.race_id AS current_race_id,
curr.handicap AS current_handicap,
curr.official_rating AS current_official_rating,
prev.scheduled_time AS previous_race_time,
prev.race_id AS previous_race_id,
prev.previous_starting_price,
prev.previous_starting_price_decimal,
prev.previous_position_in_betting,
prev.previous_finish_position,
prev.previous_unfinished,
prev.previous_distance_won,
prev.previous_distance_beaten,
prev.previous_official_rating,
prev.previous_handicap,
prev.previous_betting_text,
prev.derived_previous_odds,
prev.previous_SP_pct,
prev.previous_opening_price_pct,
-- Safe percentage movement calc using DECIMAL only
CASE
WHEN prev.previous_opening_price_pct > 0 THEN
CAST((
(CAST(prev.previous_SP_pct AS DECIMAL(20,10)) - CAST(prev.previous_opening_price_pct AS DECIMAL(20,10)))
/ CAST(prev.previous_opening_price_pct AS DECIMAL(20,10))
) * CAST(100 AS DECIMAL(20,10)) AS DECIMAL(20,10))
ELSE NULL
END AS previous_price_movement
FROM ProcessedRaces curr
LEFT JOIN ProcessedRaces prev
ON curr.runner_id = prev.runner_id
AND curr.race_type_id = prev.race_type_id
AND prev.race_rank = curr.race_rank + 1
WHERE curr.race_rank = 1
AND curr.scheduled_time >= DATE_SUB(CURRENT_DATE, INTERVAL 3 MONTH)
ORDER BY curr.race_type_id, curr.runner_id;
Extracting useful data from the betting string
By Nick Franks on Sunday, May 11th, 2025This post comes as a result of thinking about ways to calculate the price movements of horses, from the opening show to the SP. The opening show and other price movements aren’t currently available in Smartform as separate fields, however the opening show and other price movements are contained within the betting_text column in the historic_runners and historic_runners_beta tables, stored as a text string.
The question is how to split out the data. After a number of experiments, I came up with the following SQL to derive a usable price to go to work with. In my next post I will describe how to convert this to a decimal price and calculate the price movement from the opening show to starting price.
IFNULL( REPLACE( REGEXP_SUBSTR( betting_text, '(?<=op )\d{1,3}/\d{1,3}|(?<=tchd )\d{1,3}/\d{1,3}|(?<=op )Evens|(?<=tchd )Evens' ), 'Evens', '1/1' ), '0/0' ) AS derived_previous_odds
The above code looks into the string field called betting_text
and tries to find the previous odds of a horse from the point at which the betting shows open on course before any given race
Here’s what each part does:
REGEXP_SUBSTR(...)
:
This searches the text for the first match of one of the following patterns:- A fraction like
5/2
,11/8
, etc. that appears after the word “op “ (short for “opened at”) - A fraction that appears after the word “tchd “ (short for “touched”)
- The word
"Evens"
(which means 1/1 odds) after either “op ” or “tchd “
- A fraction like
REPLACE(..., 'Evens', '1/1')
:
If the match found was"Evens"
, this replaces it with"1/1"
to standardize it into fractional format.IFNULL(..., '0/0')
:
If nothing matched (i.e., there were no odds found in the text), it returns'0/0'
instead of null.
Examples
betting_text
= "op 5/1 tchd 6/1"
- Match found:
"5/1"
(after"op "
) - Replacement: None
- Final result:
"5/1"
betting_text
: "op Evens tchd 11/10"
- Match found:
"Evens"
(after"op "
) - Replacement:
"Evens"
→"1/1"
- Final result:
"1/1"
betting_text
= "op 85/40 tchd 2/1"
- Match found:
"85/40"
(after"op "
) - Replacement: None
- Final result:
"85/40"
betting_text
= "tchd 30/100 op 4/5"
- Match found:
"30/100"
(after"tchd "
) - Replacement: None
- Final result:
"30/100"
betting_text
= "non-runner, withdrawn at start"
- Match found: None (no “op” or “tchd” with odds or “Evens”)
- Replacement: Not applicable
- Final result:
"0/0"
(default value when nothing matches)
New: daily_jockeys_insights comes to Smartform
By colin on Tuesday, December 10th, 2024Hot on the heels of our post about the new daily trainers insights variables available to Smartform users, we’re pleased to announce the addition of a new corresponding table for jockeys: daily_jockeys_insights.
You can view the new schema and Smartform users can download all the data (all 2.3 million new rows) following the instructions under “Downloading the data” here.
The new table follows the format and category types designed for daily_trainers_insights, but instead curating statistics for all daily races and runners since March 2008 according to jockey performance. All the details are in the schemas, and the previous blog article on trainer_insights holds true but this time for the riders. Rather than restate the high level details in this blog post, we will instead show what it is possible to achieve using one of the unique fields in the table.
In this example, presented below, we joined the daily_jockeys_insights table to the daily_runners_insights table for all yesterday’s racing at Wolverhampton, removing non-runners, and focussed on the percentage rivals beaten (PRB) statistics for each jockey riding in each race, but over the past 5 years using the values automatically generated in the new table. The particular PRB statistic we used comes with a twist, however, in that it considers the performance of each jockey according to the handicap status in the race in which the jockey is competing. In other words, each PRB figure is applied to the jockey’s performance in handicaps or non-handicaps – and the 5 year PRB figure for all the jockeys rides up to but not including the race is presented – according to the handicap status of the current race in question. Note that each comparison, as presented below, can be generated prior to racing, and you can also interrogate previous day’s statistics by entering the relevant meeting date. We then wrote a graphics script to plot the runners in each race, showing each jockey below the runner name, and highlighting the runner and jockey in bold which has the highest PRB statistic within the context of the race. The red dashed line shows the level of the PRB for the highest rated jockey within each race. Below are the results.
Finally, let’s look at the results for each race by querying Smartform on the highlighted runners and jockeys above.
Course | Race Time | Horse | Jockey | Result | Starting Price |
Wolverhampton | 16.15 | Lewis Barnes | Kaiya Fraser | 2 | 9/4 |
Wolverhampton | 16.45 | Diomed Spirit | Marco Ghiani | 3 | 7/1 |
Wolverhampton | 17.15 | Onslow Gardens | Tyrese Cameron | 4 | 11/8 |
Wolverhampton | 17.45 | Bear Rock | R Kingscote | 2 | 66/1 |
Wolverhampton | 18.15 | Windsor Pass | Kaiya Fraser | 5 | 4/1 |
Wolverhampton | 18.45 | Eagle Day | R Kingscote | 1 | 15/2 |
Wolverhampton | 19.15 | Moon Over The Sea | Jack Doughty | 5 | 9/2 |
Wolverhampton | 19.45 | Khangai | Hector Crouch | 3 | 5/4 |
Wolverhampton | 20.15 | Alfheim | Tommie Jakes | 1 | 11/4 |
Given we are using just one variable from the new table, these are, we hope you will agree, interesting results: 2 well priced winners, with 4 placed horses also at interesting prices, and 3 losers.
What is particularly interesting about using the “by handicap status” condition is that whilst you do get some repeats (as with Richard Kingscote and Kaiya Fraser, each qualifying in 2 races, above), one jockey that has good statistics does not dominate the whole meeting’s statistics. This can happen with (for example) course statistics by jockey. Where you do want to highlight “the best” jockey at a course, this also has its uses, and this too is available in the new table, along with 40+ other variables. Enjoy!
New: daily_trainers_insights now available in Smartform
By colin on Tuesday, December 3rd, 2024We’ve added a new database table to Smartform. Over 2.29 million new rows of data and 48 columns, representing all daily runners since the daily* tables started in March 2008 (for both Flat and Jumps racing), with every runner mapped to the historic performance of the trainer responsible, with statistics aggregated for all the trainer’s runners over the previous 5 years to that run, the previous 42 days and the previous 21 days to the run, covering statistics for race type performance, race code performance, performance at distance, course, in handicaps, by age of horse and much more. Thus you can track short and long term performance for each trainer by a number of different metrics.
With Smartform, you can of course create your own statistics for historical trainer performance, and there are a practically infinite number of possibilities in addition to those included in the default table. You can see some old blog posts showing how to do this on a daily basis, here and here. However we appreciate that many users do not want to program their own statistics, maintain the data, or would like a convenient baseline of performance statistics in addition to their own work that can be queried as a table at will. For all these use cases, daily_trainers_insights is now available and can be downloaded here. See the link at the last section of the page once you are logged in to the site as a subscriber, or sign up for access if you want to become a subscriber or rejoin.
All the statistics in these tables have been engineered from the data in the Smartform database to provide insight on top of the raw data, from standard win and place strike rates to more granular variables like average number of horses beaten according to distance, course, course and distance, age, handicap, race_type and race_code. You can see the full table definition and an explanation of all the variables here.
There’s more that you can do with the new table than we have space to cover here, but we’ll be writing more about different use cases for the new table over the coming weeks. To whet the appetite, here’s a graph we threw together for today’s racing that shows off 3 of the new statistics for short, medium and long term performance statistics by percentage of rivals beaten. So each horse in each race has 3 bars for the 21 day, 42 day and 5 year strike rate by race code.
Standout short term PRB statistics for the trainer of Panama City – which of course went on to win!
Unlike most of the other tables in Smartform, there is no “historic” version of this table, since all the historic statistics for each trainer are available for every day since March 2008, as it corresponds exactly to the daily_runners_beta and daily_runners table, which also run from March 2008. However, this table contains fields that are calculated based on all previous races of the same type to give you an indication of the trainer’s historic performance and updates for every day. For example, in a flat all weather handicap race today, you will be able to find statistics relating to the trainer’s previous 5 year strike rate for all runners in flat all weather handicaps.
Since it is updated for each day you have all the statistics you need to make an informed judgement about a trainer’s historic performance for runners under today’s conditions. Similarly, you can go back in time to any previous day’s racing to find the statistics that were pertinent to the trainer’s historic performance at that time.
Each variable has been painstakingly tested to ensure that the correct previous information is provided on a “to date” basis for each runner at the time of entry in the historic database without taking into account the result of the upcoming run. This means systems backtesting and modelling use cases are all valid with this dataset.
We hope you enjoy using daily_trainers_insights as much as we have enjoyed crafting it and writing about it. If you have questions about the new table, please do not hesitate to get in touch.
Nine (9) new fields for Smartform
By colin on Friday, November 15th, 2024We’ve just updated daily_runners_insights and historic_runners_insights. These tables already contain numerous utility or short cut fields that mean users can avoid lengthy queries on each runner in a race to find details on their previous performances and instead query the race runners and have all that data in a single row, for immediate analysis.
The recent updates fill out those details for a few variables that were previously missing for the previous few races, such as the course last time out, the race type and the jockey. We have jockey changes, course changes and race type changes – all themselves useful as rapid indicators for differences since the last run, but now we can be more specific. Check out the table schemas, linked above, for full details.
Stay tuned for a number of (even more) exciting additions to Smartform in the coming weeks!
Roger Fell : More trainer_ids to merge
By Henry Young on Thursday, October 24th, 2024Last year I wrote about Mark Johnston partnering with then handing over his trainers license to his son Charlie and the impact this had on Smartform’s trainer_id
. Those of us who like to aggregate data by trainer_id
, for example to generate trainer strike rates, might wish to acknowledge that the Johnstons’ yard and training methods remained substantially the same through this handover of the reins (pun intended) by merging all the various trainer_id
values together into one. Well, it’s happened again …
Roger Fell’s yard has been going through some drama with the addition of Sean Murray as a partner on the trainer’s license. However Sean’s name has more recently disappeared from the license. Roger Fell continues in his own right, an example to all of us, still working at age 70. One consequence is that Smartform’s data provider has changed trainer_id
, but only when Sean Murray was dropped, not when he was added. We can observe this with the following query:
select distinct trainer_id, trainer_name from historic_runners_beta where trainer_name like "%Fell%";
+------------+--------------------------+
| trainer_id | trainer_name |
+------------+--------------------------+
| 1220 | R Fell |
...
| 128659 | Roger Fell |
| 128659 | Roger Fell & Sean Murray |
| 161959 | Roger Fell |
+------------+--------------------------+
5 rows in set (6.30 sec)
Note there is an unrelated trainer whose name contains “Fell” whom I have removed from the results table for clarity.
The first thing we see is that when Sean joined the trainer license, the trainer_id
remained the same. But when Sean left the license, a new trainer_id
was created for Roger on his own. If you take the view that nothing significant has changed about how the yard is run, the staff, the training methods, etc, you may prefer to see results from all four Fell licenses under a single trainer_id
. This can be done with the following SQL script:
SET SQL_SAFE_UPDATES = 0;
UPDATE historic_runners_beta SET trainer_id = 161959 WHERE trainer_name = "R Fell";
UPDATE historic_runners_beta SET trainer_id = 161959 WHERE trainer_name = "Roger Fell";
UPDATE historic_runners_beta SET trainer_id = 161959 WHERE trainer_name = "M Johnston";
SET SQL_SAFE_UPDATES = 1;
Note that here we are using the newest trainer_id
to tag all past results. This means the modification is a one-time deal and all future runners/races will continue to use the same trainer_id
. If we had used any of the older trainer_id
values, we would have to apply the fix script every day, which would be far less convenient.
This concept is easily extended to other trainer changes. For example, if your would like aggregated stats for the partnership between Rachel Cook and John Bridger to be merged with John’s longer standing stats, you can add the following to your script:
UPDATE historic_runners_beta SET trainer_id = 157470 WHERE trainer_name = "J J Bridger";
If you find there are other trainer name changes that have resulted in a new trainer_id
which you would prefer to see merged, you can apply similar techniques.
If you know of other cases of trainer name changes which represent a continuation of substantially the same yard, please feel free to comment …
Ok, this doesn’t happen every day, but when it does…
By colin on Thursday, October 3rd, 2024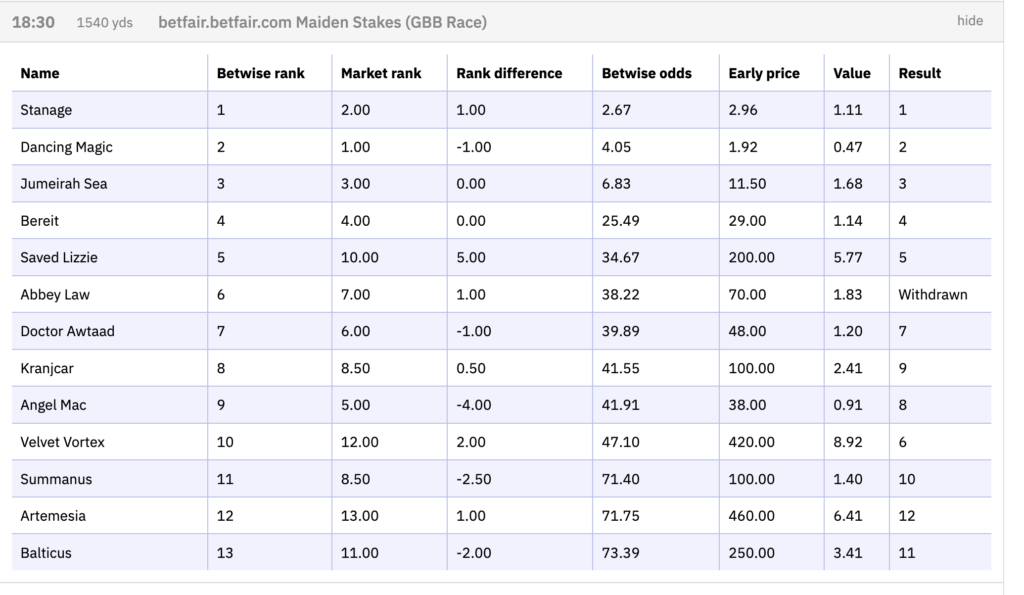
In the screenshot bove, we see key metrics from the Betwise Daily Oddsline service. Here’s a quick breakdown of what each represents:
- Name: The horse’s name.
- Betwise rank: A ranking assigned by the Betwise algorithm based on the horse’s predicted chances of winning.
- Market rank: The ranking according to the betting market’s early prices.
- Rank difference: The difference between Betwise rank and market rank, highlighting where Betwise disagrees with the market.
- Betwise odds: The odds predicted by the Betwise model, reflecting its calculation of each horse’s winning probability.
- Early price: The early market odds available on Betfair.
- Value: The ratio of early price to Betwise odds, showing potential value for bettors.
- Result: The horse’s actual finishing position. (Of course, when odds are posted before the race, this column is empty! But after the result is updated, nothing changes.)
This race was run just a few hours ago, and while we don’t see outcomes this perfect every time, the Betwise rankings aligned almost perfectly with the race result, right to the smallest places. You’d not have made much money with this prediction, but it’s notable that Saved Lizzie, ranked 5th by the oddsline, was only ranked 10th by the market, hence a big price discrepancy, almost 6 times the Betwise price, despite finishing exactly where predicted, in 5th place.
The strong correlation between the Betwise rank and actual race outcomes highlights the power of the Oddsline’s approach. By leveraging statistical models and machine learning, it captures the critical factors needed to provide value without relying on the market. As we discussed in this week’s earlier blog post, this data-driven method offers insights you won’t find elsewhere.
If you’re looking to sharpen your betting strategy with a data-driven perspective, you can sign up for the Betwise Oddsline here.
Harnessing Machine Learning for Smarter Market Insights with the Betwise Odds Line
By colin on Wednesday, October 2nd, 2024The modern horse racing landscape has evolved significantly with the introduction of technology-driven tools that enable bettors and analysts to make more informed decisions. 15 years ago we wrote about how to harness the value of a machine learning driven oddsline in the book Automatic Exchange Betting. For over four years, we offered a free daily oddsline for all types of thoroughbred horse racing in the UK and Ireland. We’ve been continually improving the Betwise Odds Line, and with the latest version—now available as a low-cost subscription service—we have developed an advanced, machine learning-driven tool that not only offers a solid foundation for race analysis but also offers a form driven perspective on the marke. As we continue to refine and enhance this tool, our mission with the oddsline is to help subscribers identify opportunities and navigate the more uncertain areas of the betting landscape.
The Betwise Odds Line is powered by the SmartForm database. The database itself provides all the raw data needed to build your own models, and we use it ourselves for the oddsline process. That process begins with feature engineering, and we have now created and tested a feature universe of over 700 derived variables for this purpose. These variables cover every conceivable angle, from a horse’s past performance to trainer, jockey and sire statistics as well as our own proprietary speed ratings, creating a rich dataset that drives our machine learning algorithms. Users of the Smartform database are able to do this for themseleves and in many cases build their own models. In our case, this comprehensive data set goes into models aimed at predicting the probability of a horse winning a race. The objective is to enable the Betwise Odds Line to offer a consistent, objective, and powerful approach to understanding race probabilities. By training on this vast amount of information, our model can rank horses in terms of probability of winning, with forecast probabilities or odds for each contender. This data – the oddsline – can then be used to identify where the market might be overestimating or underestimating a contender’s chances, or indeed where the market clearly diverges with the oddsline, and may “know” something pure form cannot reveal. This makes the odds line not just a foundation, but a strategic tool that can reveal genuine opportunities and those horses with the strongest probabilities of winning any given race. Beyond that, since this is not a “tipster” service, we can look at the structure of any given market versus the oddsline, and consider how the ranking order produced by the oddsline can be used to identify value in place bets, forecasts, tricasts and multiples – or pool bets, such as the placepot.
While the Betwise Odds Line excels in providing a robust data-driven foundation, we are completely aware of the inherent uncertainties in predicting outcomes. There are always factors that form data cannot fully capture — the form database has no knowledge of home gallops, horse improvement or deterioration off the track, trainers and owners “plots”, horses acting up on the way to the post, the unpredictable nature of the race itself and so on – all of which will alter the dynamics of the race and the probability that any horse has of winning. These are the nuances that make betting both challenging and intriguing, and this uncertainty will often be mirrored in fluctuating market odds and rankings. Our ongoing development efforts focus on highlighting where the odds line’s predictions might be more or less confident, and it’s important to note that the oddsline does not use any market information. This opens up new avenues for understanding how market pricing compares to raw race probabilities. In fact, both the more confident predictions and the fuzzier areas can present opportunities. By understanding where the odds line is most accurate and where it’s more uncertain, as well as where the market may be more influential, bettors can tailor their strategies accordingly, exploring these subtleties to find value that others might miss.
Since launching the Betwise Odds Line, we’ve now added live results, allowing you to track performance in real time. We will soon be adding tote returns and live pools, enhancing the ability to use the oddsline for deeper race analysis, particularly where ranking order within the race is paramount for exotic bet strategies. Not to mention providing rankings to help navigate a whole meeting.
As we continue to refine the service, our goal is to make it an indispensable tool for bettors. By subscribing, you’re not just gaining access to a an oddsline—you’re joining a service dedicated to exploring the complexities of horse racing and probability. Our journey is about more than just offering probabilities; it’s about understanding the dynamics of horse racing markets and providing you with the insights to make smarter, more informed decisions. Whether you’re seeking clear-cut value or eager to explore the sport’s fascinating uncertainties, join us as we push the boundaries of data-driven insights for smarter market strategies.
You can find out more here and sign up at: Betwise Odds Line.
Introducing GitHub
By Henry Young on Tuesday, May 14th, 2024Betwise has created an area on GitHub for Smartform community members to share their ideas, code, etc:
https://github.com/Betwise-Smartform
GitHub is one the most popular sites for hosting open source software and other types of shared information. I have kicked things off by uploading a simplified version of a race card tool I built using Excel/VBA:
https://github.com/Betwise-Smartform/ExcelCard
In my next blog post I’ll outline some of the techniques used to get data from Smartform into Excel using VBA (Visual Basic for Applications) to query the database. Then in subsequent blog posts I’ll add a variety of interesting predictive statistics columns that will turn the race card into a handy tool for analyzing races.
Eventually we’ll end up with the race card that I use daily to inform my own betting selections. Here’s an example of the 15:50 at Haydock on 7 June 2020, won by Golden Melody with Velocistar coming in last. My selection was to lay Velocistar. I won’t go into the details of what these columns are just yet, but a quick eyeball of the various numbers (mostly % strike rates aggregated in various ways) shows big numbers for Golden Melody and small numbers for Velocistar, suggesting back and lay opportunities respectively. Click on the image to blow it up.
As we progress through the series, I hope this will inspire you to try out some of the ideas, contribute back your own improvements and even create new GitHub repositories yourself to share your own work with the Smartform community.
Pat Cosgrave’s 28 Day Ban
By Henry Young on Saturday, April 22nd, 2023You may have seen that Pat Cosgrave received a 28 day ban for easing what should have been an easy winner on Concord in the Chelmsford 8pm on 20th April 2023. You can read the Racing Post report here:
Of immediate interest to me is why did he do this? While some may suggest dubious intent, my first thought was that as a seasoned jockey, and given the insights into jockey changing room culture that leak out from time to time, perhaps he simply enjoys underlining his seniority there with over the shoulder glances and a “look at me winning with no hands” attitude which he simply misjudged on this occasion. So I wanted to re-watch some of his other big distance wins to assess his riding manner in similar situations.
But how to find these easy win races ?
Smartform to the rescue with a simple SQL query:
mysql> select scheduled_time, name, course, finish_position as position, distance_won as distance from historic_runners_beta join historic_races_beta using (race_id) where jockey_name = "P Cosgrave" and finish_position = 1 order by distance_won desc limit 20;
+---------------------+--------------------+---------------+----------+----------+
| scheduled_time | name | course | position | distance |
+---------------------+--------------------+---------------+----------+----------+
| 2022-07-07 17:53:00 | Caius Chorister | Epsom_Downs | 1 | 19.00 |
| 2006-07-18 19:10:00 | Holdin Foldin | Newcastle | 1 | 17.00 |
| 2003-10-25 17:00:00 | Excalibur | Curragh | 1 | 15.00 |
| 2015-05-20 14:30:00 | Wonder Laish | Lingfield | 1 | 15.00 |
| 2015-10-06 14:10:00 | Light Music | Leicester | 1 | 14.00 |
| 2009-01-29 14:20:00 | More Time Tim | Southwell | 1 | 13.00 |
| 2018-07-19 14:20:00 | Desert Fire | Leicester | 1 | 12.00 |
| 2008-12-12 12:55:00 | Confucius Captain | Southwell | 1 | 10.00 |
| 2010-04-27 16:10:00 | On Her Way | Yarmouth | 1 | 10.00 |
| 2018-09-22 15:55:00 | Stars Over The Sea | Newmarket | 1 | 10.00 |
| 2012-10-25 16:40:00 | Saaboog | Southwell | 1 | 9.00 |
| 2006-03-31 15:40:00 | John Forbes | Musselburgh | 1 | 8.00 |
| 2015-05-20 15:00:00 | I'm Harry | Lingfield | 1 | 8.00 |
| 2018-06-17 15:10:00 | Pirate King | Doncaster | 1 | 8.00 |
| 2019-05-19 17:30:00 | Great Example | Ripon | 1 | 8.00 |
| 2004-07-19 18:30:00 | Orpailleur | Ballinrobe | 1 | 7.00 |
| 2007-07-04 17:35:00 | Serena's Storm | Catterick | 1 | 7.00 |
| 2008-01-07 13:50:00 | Home | Southwell | 1 | 7.00 |
| 2014-09-06 20:50:00 | Fiftyshadesfreed | Wolverhampton | 1 | 7.00 |
| 2015-05-16 13:45:00 | Firmdecisions | Newmarket | 1 | 7.00 |
+---------------------+--------------------+---------------+----------+----------+
20 rows in set (5.55 sec)
You can watch the top race here, with no apparent easing but plenty of glances behind:
The point of this article is really to emphasize that with Smartform and a modicum of SQL knowledge, you can easily answer those oddball questions that come up from time to time, that no existing software solution or website will answer as easily.